일 | 월 | 화 | 수 | 목 | 금 | 토 |
---|---|---|---|---|---|---|
1 | 2 | 3 | 4 | 5 | ||
6 | 7 | 8 | 9 | 10 | 11 | 12 |
13 | 14 | 15 | 16 | 17 | 18 | 19 |
20 | 21 | 22 | 23 | 24 | 25 | 26 |
27 | 28 | 29 | 30 |
- DP
- Python
- Vit
- diffusion models
- 코테
- VirtualTryON
- image editing
- Programmers
- video generation
- segmentation map
- video editing
- diffusion model
- segmenation map generation
- diffusion
- flow matching
- 3d generation
- BOJ
- flipd
- rectified flow
- inversion
- 프로그래머스
- transformer
- 네이버 부스트캠프 ai tech 6기
- masactrl
- 논문리뷰
- 코딩테스트
- noise optimization
- 3d editing
- memorization
- visiontransformer
- Today
- Total
평범한 필기장
[평범한 학부생이 하는 논문 리뷰] Denoising Diffusion Probabilistic Models (DDPM) 본문
[평범한 학부생이 하는 논문 리뷰] Denoising Diffusion Probabilistic Models (DDPM)
junseok-rh 2024. 1. 18. 00:24이번에 리뷰할 논문은 그 유명한! DDPM! Diffusion의 기초 논문들은 확실하게 이해하고 넘어가는 것이 좋다는 멘토님의 조언에 따라 이번 ddpm도 시간은 오래 걸리겠지만 최대한 꼼꼼하게 읽어서 자세히 리뷰하는 것을 목표로 포스팅을 할 것이다. 이전 NCSN논문도 꼼꼼하게 했다고 생각하지만 아직 100프로 이해했다고 자부할 수 없었다. 그래서 ddpm도 100프로는 아니지만 70,80프로를 넘어 90프로는 이해하자는 목표로 공부했다.
https://arxiv.org/pdf/2006.11239.pdf
그럼 이제 논문 리뷰를 시작하겠다.
1. Introduction
이 논문에서는 diffusion probablilistic model (diffusion model)의 진전을 소개한다고 한다. Diffusion model은 유한한 시간 후에 데이터와 일치하는 샘플을 생성하기 위해 variational inference을 사용하여 훈련된 매개변수화된 마르코프 체인이다. 이 체인의 transitions은 신호가 파괴될 때까지 샘플링의 반대 방향으로 데이터에 점차적으로 노이즈를 추가하는 마르코프 체인인 diffusion process를 reverse하도록 학습된다.
이전까지 diffusion모델이 고품질의 샘플을 생성할 수 있다고 입증된적 없지만 이 논문에서는 diffusion model이 실제로 고품질 샘플을 생성할 수 있으며, 때로는 다른 유형의 생성 모델보다 더 나은 결과를 생성할 수 있음을 보여준다. 또한 diffusion model의 특정 parameterization가 훈련 중 여러 노이즈 레벨에 걸친 denoising score matching과 샘플링 중 annealed Langevin dynamics와 동등하다는 것을 보여준다. 이 parameterization으로 최상의 샘플 quality를 얻었으므로 이 동등성이 주요 기여 중 하나라고 한다.
논문에서 모델의 lossless codelength 대부분이 감지할 수 없는 이미지 detail을 설명하는 데 사용된다는 것을 발견했다고 한다.
2. Background
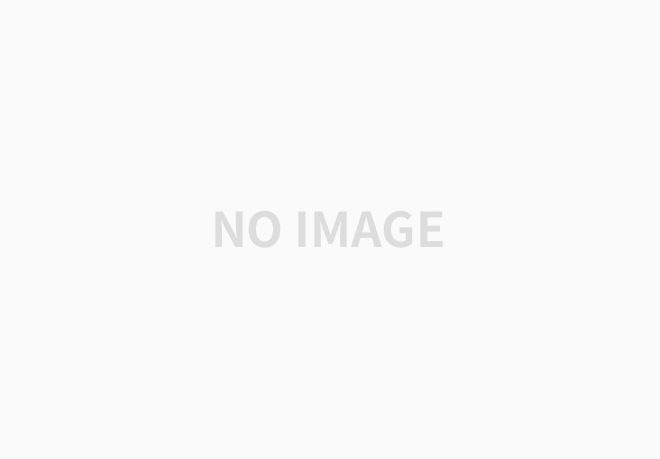
2.1 Forward(Diffusion) Process
Diffusion model은 위 이미지에서 볼 수 있듯이 두가지 process가 존재한다. 먼저 두 process중에서 forward(diffusion) process부터 알아보자. 이 process는 말 그대로 이미지에 노이즈를 주입해주는 process이다. 이미지 x0에 가우시안 노이즈를 점점 추가해줌으로써 최종적으로 완전한 가우시안 노이즈 xT∼N(xT;0,I)를 얻게 된다.
q(x1:T|x0):=TΠt=1q(xt|xt−1),q(xt|xt−1):=N(xt;√1−βtxt−1,βtI),(2)
여기서 t−1시점에서 노이즈를 추가해서 t시점의 분포 q(xt|xt−1)를 얻기에 Markov Chain이다.
또한 q(xt|xt−1):=N(xt;√1−βtxt−1,βtI)로 두는 이유는 xt=√1−βtxt−1+√βtz, where z∼N(0,I)에서 xt의 분산이 너무 커지는 것을 방지하기 위함이라고 한다.
2.2 Reverse Process
Forward Process를 통해 이미지를 완전한 노이즈로 만들었으면 우리는 그 반대 방향인 노이즈를 지우는 reverse process를 알아내야한다. 우리가 알고싶은 것은 q(xt−1|xt)이다. 하지만 이를 알기 힘드므로 approximation인 pθ(xt−1|xt)를 구하는 것이 목적이다. Reverse process는 아래와 같이 정의됐다.
pθ(x0:T):=p(xT)TΠt=1pθ(xt−1|xt),pθ(xt−1|xt):=N(xt−1;µθ(xt,t),Σθ(xt,t)),(1)
Reverse process또한 forward process와 마찬가지의 이유로 Markov Chain이다. 또한 완전한 노이즈 p(xT)=N(xT;0,I)에서 시작한다. βt가 작으면 q(xt|xt−1)와 q(xt−1|xt)가 같은 형태를 띈다고 증명이 됐기 때문에 pθ가 가우시안 분포이라고 할 수 있다.
2.3 Objective Function
Diffusion Model은 pθ(x0):=∫pθ(x0:T)dx1:T로 정의됐고, 학습은 pθ(x0)의 negative log likelihood(NLL)의 variational bound를 이용한다. 아래와 같이 diffusion model의 objective funcion이 유도되는 것을 볼 수 있다.
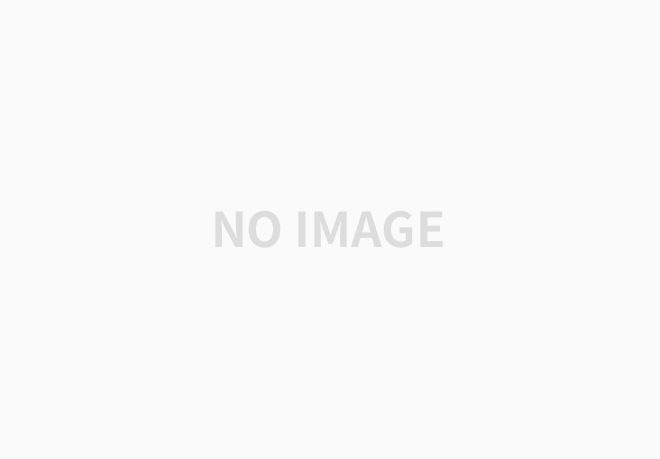
앞서 우리는 forward process q(xt|xt−1)의 반대인 q(xt−1|xt)를 알고 싶다고 했고 그를 approximate하는 pθ(xt−1|xt)를 찾고자했다. 하지만 q(xt−1|xt)는 intractable하기 때문에 이와 직접 비교해서 KL divergence를 구할 수는 없다. 하지만 q(xt−1|xt)대신 q(xt−1|xt,x0)는 tractable하기 때문에 이와 KL divergence를 줄이는 방식으로 학습이 가능하다.
Forward process는 q(xt|xt−1):=N(xt;√1−βtxt−1,βtI)로 정의됐다. 야래와 같은 방법으로 x0에서 xt로 바로 건너 뛸수가 있다. 이 성질을 이용해서 x0에 condition된 backward process posterior q(xt−1|xt,x0)를 아래와 같이 구할 수 있다.
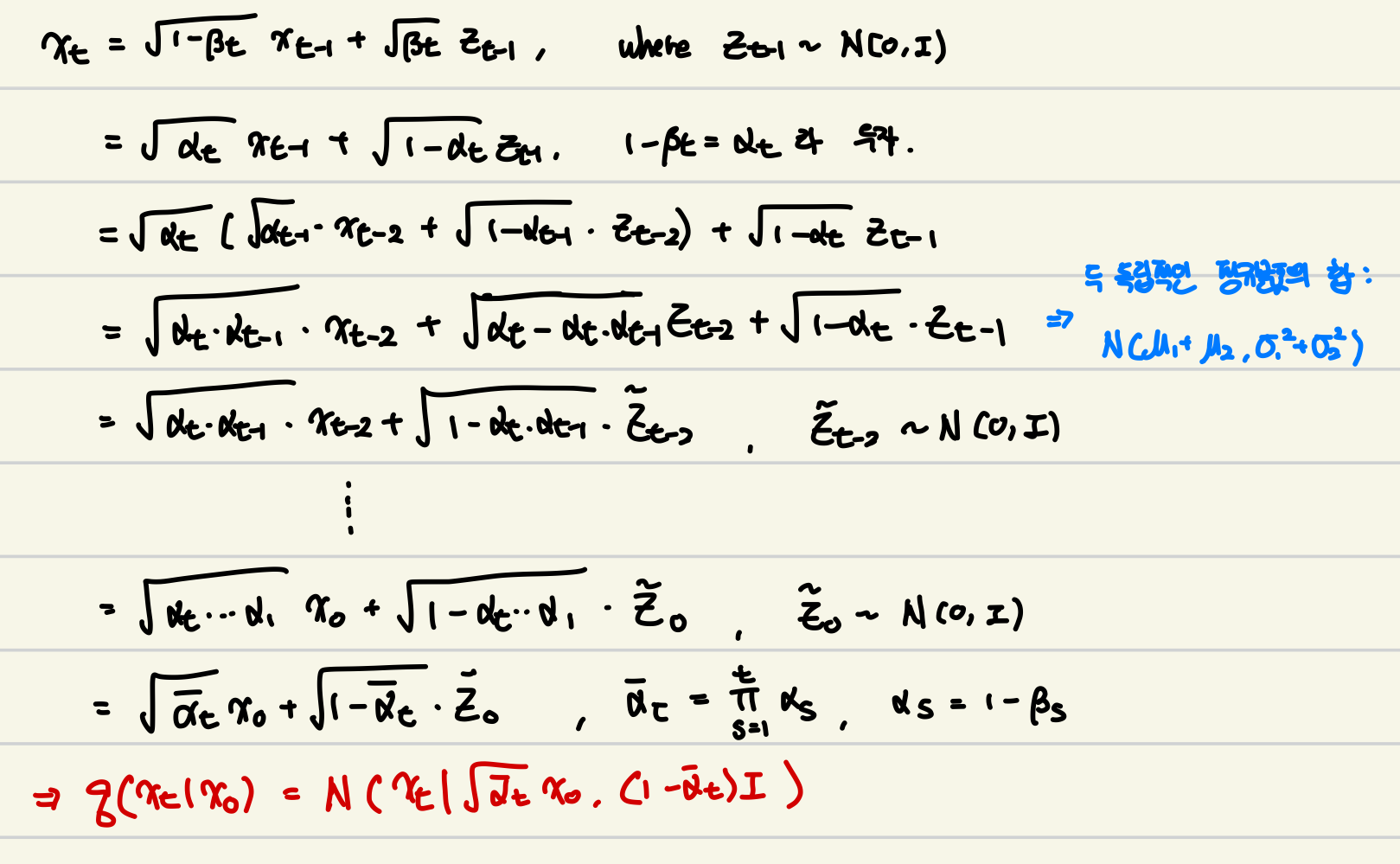

위의 결론으로 아래의 식이 나온다. 아래의 식(6)을 논문에서는 forward process posterior라고 한다.
q(xt−1|xt,x0)=N(xt−1;˜µt(xt,x0),˜βtI),(6)
여기서 ˜µt(xt,x0):=√ˉαt−1βt1−ˉαtx0+√αt(1−ˉαt−1)1−ˉαtxt 및 ˜βt:=1−ˉαt−11−ˉαtβt,(7)
3 Diffusion models and denoising autoencoder
이번 섹션에서 objective function에서 각 term에 대해 알아보자.
Eq[DKL(q(xT|x0)‖p(xT))⏟LT+Σt>1DKL(q(xt−1|xt,x0)‖pθ(xt−1|xt))⏟Lt−1−logpθ(x0|x1)⏟L0],(5)
LT는 VAE의 loss에서 regularization term과 상응하고, Lt−1은 denoising process, L0은 VAE의 loss에서 reconstruction term과 상응한다.
3.1 Forward process and LT
논문에서 p(xT)=N(xT;0,I)으로 고정되어있고, q(xT|x0)=N(xt;√ˉαtx0,(1−ˉαt)I)으로 learnable parameter가 없기 때문에 학습중에는 상수로 고정된다.
3.2 Reverse process and L1:T−1
Lt−1=DKL(q(xt−1|xt,x0)‖pθ(xt−1|xt))이고 q(xt−1|xt,x0)=N(xt−1;˜µt(xt,x0),˜βtI), pθ(xt−1|xt)=N(xt−1;µθ(xt,t),Σθ(xt,t))이다. pθ에서 μθ와 Σθ둘다 모수 θ를 포함해서 learnable parameter이다.
- Σθ
논문에서 Σθ를 임의의 상수인 σ2tI로 두었다. σ2t=βt와 σ2t=˜βt=1−ˉαt−11−ˉαtβt 로 모두 사용가능하다고 한다.
- µθ(xt,t)
pθ(xt−1|xt)=N(xt−1;µθ(xt,t),σ2tI)를 사용하면 다음과 같이 쓸 수 있다:(여기서 σ2t를 ˜βt로 두면 pθ와 q의 분산이 같아진다.)
Lt−1=DKL(q(xt−1)|xt,x0)‖pθ(xt−1|xt))=Eq[12σ2t‖˜µt(xt,x0)−µθ(xt,t)‖2]+C,(8)
여기서 C는 θ에 의존하지 않는 상수이고 ˜μt는 q(xt−1|xt,x0)의 평균이다.
따라서 ˜µt를 예측하는 모델이라는 것을 알 수 있다.
- ϵθ
q(xt|x0)=N(xt;√ˉαtx0,(1−ˉαt)I)를 xt(x0,ϵ)=√ˉαtx0+√1−ˉαtϵ for ϵ∼N(0,I)로 reparameterize하고 forward 프로세스 posteriors 식 (7)을 적용하면 방정식 (8)을 더 확장할 수 있다.
Lt−1−C=Eq[12σ2t‖˜µt(xt,x0)−µθ(xt,t)‖2]에서 xt∼q(xt|x0)이므로 xt=√ˉαtx0+√1−ˉαtϵ, where ϵ∼N(0,I)로 표현할 수 있다. 이를 ˜μ에 x0에 대입을 하고 정리를 하면 ˜μt=1√αt(xt−βt√1−ˉαtϵ)이 된다.
이를 통해 식(8)을 다시 표현하면 아래와 같은 식이 나온다.
Lt−1−C=Ex0∼q(x0),ϵ∼N(0,I)[12σ2t‖1√αt(xt−βt√1−ˉαtϵ)−μθ(xt,t)‖2](10)
위 식을 보면 μθ가 1√αt(xt−βt√1−ˉαtϵ)를 예측하게 되는 것이고,
μθ=1√αt(xt−βt1−ˉαtϵθ(xt,t))(11)
로 parameterize할 수 있다. 결국 우리의 backward model은 xt−1∼pθ(xt−1|xt)=N(xt−1;μθ(x,t),σ2tI)이 된다. 그래서 우리는 algorithm 2에서 나온 것 처럼 샘플링을 할 수 있다.
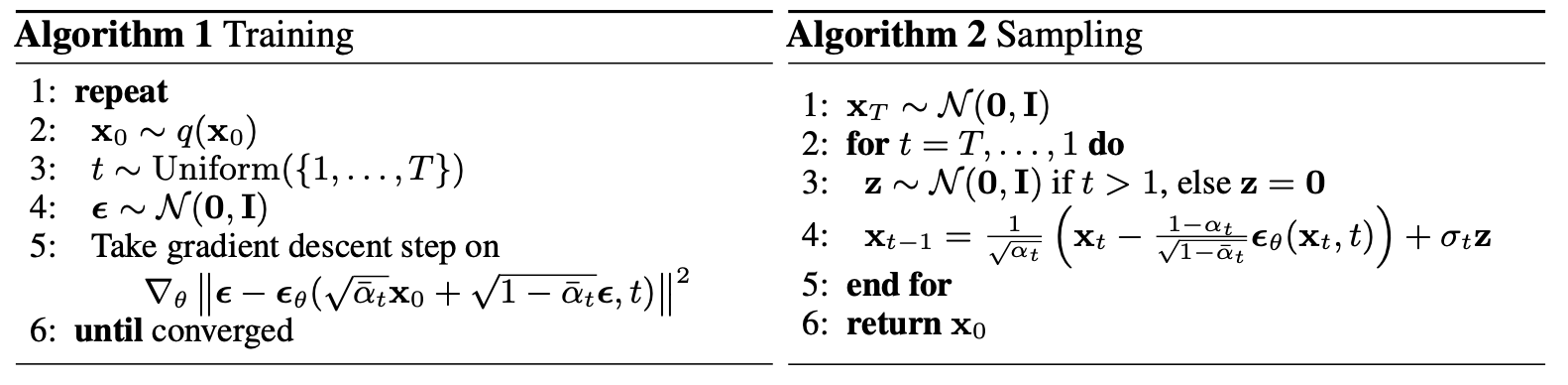
식 (11)을 (10)에 넣고 q(xt|x0)=N(xt;√ˉαtx0,(1−ˉαt)I)를 이용하면 아래와 같이 식을 정리할 수 있다.
Ex0∼q(x0),ϵ∼N(0,I)[β2t2σ2tαt(1−ˉαt)‖ϵ−ϵθ(√ˉαtx0+√1−ˉαtϵ,t)‖2],(12)
Reverse process 평균 함수 근사치 µθ를 훈련하여 ˜µt 를 예측하거나 parameterize를 수정하여 ϵ을 예측하도록 훈련할 수 있다. ϵ - predict parameterization은 Langevin dynamics와 유사하며, denoising score matching과 유사한 목표에 대한 diffusion 모델의 variational bound를 단순화한다는 것을 보여준다.
실험을 통해, 아래와 같이 계수를 없앤 단순화한 loss가 더 품질이 우수한 이미지를 생성하여 최종적으로는 아래의 loss 함수를 쓴다고 한다.
Lsimple(θ):=Et,x0,ϵ[‖ϵ−ϵθ(√ˉαtx0+√1−ˉαtϵ,t)‖2(14)
3.3 Data scaling, reverse process decoder, and L0
Reverse process를 통해 나온 x1을 실제 데이터 분포인 q(x0)의 domain으로 보내줘야한다. 이 분포를 independent discrete decoder로 한다.
pθ(x0|x1)=DΠi=1∫δ+(xi0)δ−(xi0)N(x;µiθ(x1,1),σ21)dx
δ+(x)={∞if x=1x+1255if x<1δ−(x)={−∞if x=−1x−1255if x>−1(13)
여기서 D는 데이터 차원이고 i 윗첨자는 한 좌표의 추출을 나타낸다.
VAE loss에서의 reconstruction loss와 같은 부분인데 이미지 x0에서 노이즈가 조금 들어간 x1과 x0의 MSE loss를 최소화 하는 것과 같다고 한다.
위 과정을 통해,
LossDiffusion=Eq[DKL(q(xT|x0)‖p(xT))⏟LT+Σt>1DKL(q(xt−1|xt,x0)‖pθ(xt−1|xt))⏟Lt−1−logpθ(x0|x1)⏟L0]
이 논문에서는 위 diffusion의 loss함수를 아래와 같이 단순화 했다.
LossDDPM=Et,x0,ϵ[‖ϵ−ϵθ(√ˉαtx0+√1−ˉαtϵ,t)‖2
이러한 점이 이 논문의 가장 큰 contribution이라고 한다.
4. Experiments
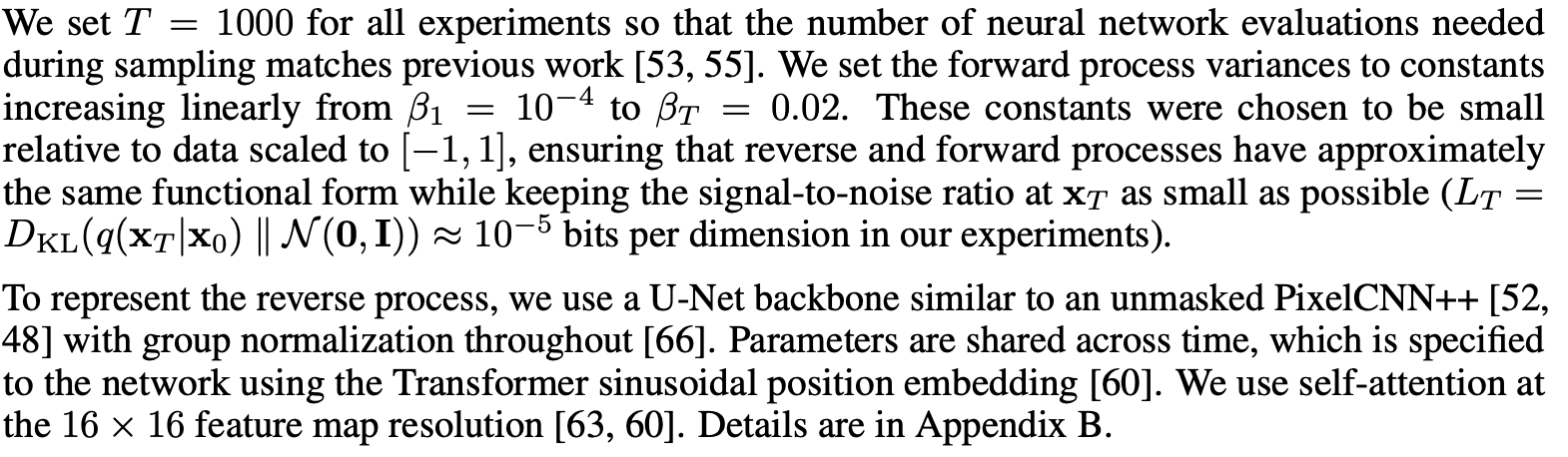
실험 설정은 위와 같고 더 자세한 설명은 부록을 참고하면 된다.
4.1 Sample quality & Reverse process parameterization and training objective ablation

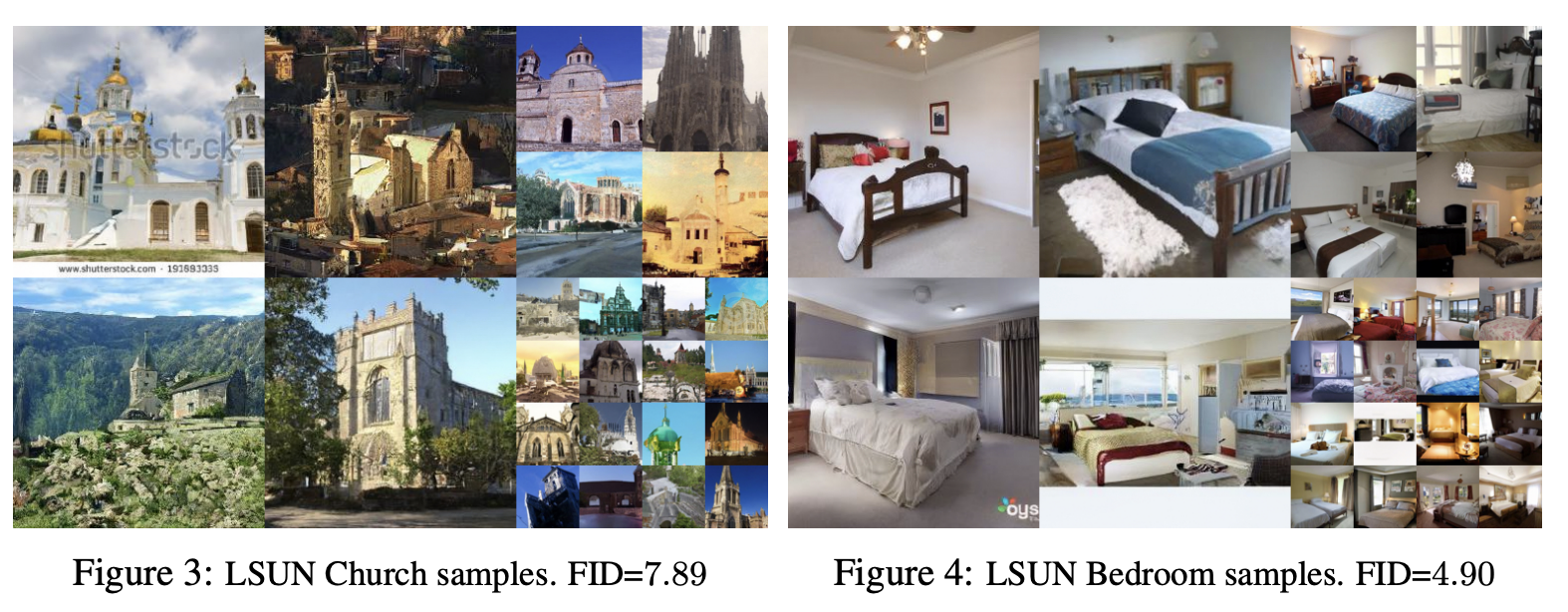
표 2의 결과를 보면 논문에서 제안한 ϵ 예측은 고정 분산으로 variational bound에 대해 훈련할 때 ˜µ를 예측하는 것과 거의 비슷한 성능을 보이지만, 단순화된 목표로 훈련할 때 훨씬 더 나은 성능을 보인다.
Diffusion 두번 째 논문인 DDPM논문 리뷰를 이렇게 마쳤는데 이번에도 최대한 자세하게 하려고 노력했다. 하지만 역시 모르는게 많았고 스터디를 진행하면서 모르는 것들을 채워야겠다.... 이번 논문도 자세하게 하려다 보니 길이가 길어진 것 같은데 긴 포스팅을 읽어주셔서 너무 감사합니다!
QnA
-> 스터디 진행 후에 추가할 예정!