일 | 월 | 화 | 수 | 목 | 금 | 토 |
---|---|---|---|---|---|---|
1 | 2 | 3 | ||||
4 | 5 | 6 | 7 | 8 | 9 | 10 |
11 | 12 | 13 | 14 | 15 | 16 | 17 |
18 | 19 | 20 | 21 | 22 | 23 | 24 |
25 | 26 | 27 | 28 | 29 | 30 | 31 |
- image editing
- 논문리뷰
- 코딩테스트
- diffusion model
- memorization
- 코테
- rectified flow
- video editing
- image generation
- Vit
- diffusion models
- BOJ
- ddim inversion
- visiontransformer
- freeinv
- 3d editing
- flow matching models
- Programmers
- video generation
- 3d generation
- DP
- one step generation
- 네이버 부스트캠프 ai tech 6기
- Python
- diffusion
- transformer
- inversion
- VirtualTryON
- 프로그래머스
- shortcut model
- Today
- Total
목록AI (65)
평범한 필기장
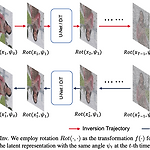
https://yuxiangbao.github.io/FreeInv/ FreeInv: Free Lunch for Improving DDIM InversionNaive DDIM inversion process usually suffers from a trajectory deviation issue, i.e., the latent trajectory during reconstruction deviates from the one during inversion. To alleviate this issue, previous methods either learn to mitigate the deviation or deyuxiangbao.github.ioAbstract Naive DDIM은 reconstruction과..
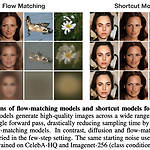
paper : https://arxiv.org/abs/2410.12557 One Step Diffusion via Shortcut ModelsDiffusion models and flow-matching models have enabled generating diverse and realistic images by learning to transfer noise to data. However, sampling from these models involves iterative denoising over many neural network passes, making generation slow aarxiv.orgAbstract본 논문은 shortcut model을 제안한다. 이는 single network를..
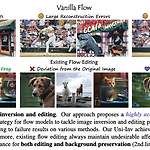
Paper : https://arxiv.org/abs/2504.13109 UniEdit-Flow: Unleashing Inversion and Editing in the Era of Flow ModelsFlow matching models have emerged as a strong alternative to diffusion models, but existing inversion and editing methods designed for diffusion are often ineffective or inapplicable to them. The straight-line, non-crossing trajectories of flow models posearxiv.orgAbstractDiffusion mo..
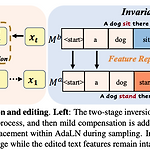
Paper : https://arxiv.org/abs/2411.15843 Unveil Inversion and Invariance in Flow Transformer for Versatile Image EditingLeveraging the large generative prior of the flow transformer for tuning-free image editing requires authentic inversion to project the image into the model's domain and a flexible invariance control mechanism to preserve non-target contents. However, thearxiv.org1. Introductio..
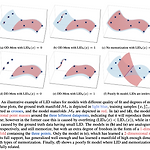
Paper : https://arxiv.org/abs/2411.00113 A Geometric Framework for Understanding Memorization in Generative ModelsAs deep generative models have progressed, recent work has shown them to be capable of memorizing and reproducing training datapoints when deployed. These findings call into question the usability of generative models, especially in light of the legal andarxiv.orgAbstract본 논문은 memori..
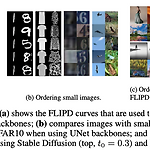
Paper : https://arxiv.org/abs/2406.03537 A Geometric View of Data Complexity: Efficient Local Intrinsic Dimension Estimation with Diffusion ModelsHigh-dimensional data commonly lies on low-dimensional submanifolds, and estimating the local intrinsic dimension (LID) of a datum -- i.e. the dimension of the submanifold it belongs to -- is a longstanding problem. LID can be understood as the number ..
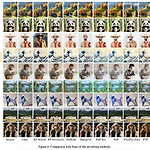
Paper : https://arxiv.org/abs/2412.07517 FireFlow: Fast Inversion of Rectified Flow for Image Semantic EditingThough Rectified Flows (ReFlows) with distillation offers a promising way for fast sampling, its fast inversion transforms images back to structured noise for recovery and following editing remains unsolved. This paper introduces FireFlow, a simple yet effarxiv.orgAbstract Rectified Flow..
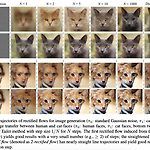
Paper : https://arxiv.org/abs/2209.03003 Flow Straight and Fast: Learning to Generate and Transfer Data with Rectified FlowWe present rectified flow, a surprisingly simple approach to learning (neural) ordinary differential equation (ODE) models to transport between two empirically observed distributions π_0 and π_1, hence providing a unified solution to generative modelingarxiv.orgAbstract 본 논문..
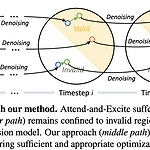
Paper : https://arxiv.org/abs/2404.04650 InitNO: Boosting Text-to-Image Diffusion Models via Initial Noise OptimizationRecent strides in the development of diffusion models, exemplified by advancements such as Stable Diffusion, have underscored their remarkable prowess in generating visually compelling images. However, the imperative of achieving a seamless alignment betwearxiv.orgAbstract 모든 ra..
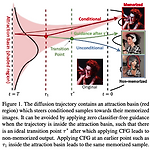
Paper : https://arxiv.org/abs/2411.16738 Classifier-Free Guidance inside the Attraction Basin May Cause MemorizationDiffusion models are prone to exactly reproduce images from the training data. This exact reproduction of the training data is concerning as it can lead to copyright infringement and/or leakage of privacy-sensitive information. In this paper, we present aarxiv.orgAbstract해결하려는 문제 D..