일 | 월 | 화 | 수 | 목 | 금 | 토 |
---|---|---|---|---|---|---|
1 | 2 | 3 | 4 | |||
5 | 6 | 7 | 8 | 9 | 10 | 11 |
12 | 13 | 14 | 15 | 16 | 17 | 18 |
19 | 20 | 21 | 22 | 23 | 24 | 25 |
26 | 27 | 28 | 29 | 30 | 31 |
- 코딩테스트
- segmenation map generation
- image editing
- Vit
- Programmers
- 코테
- VirtualTryON
- controllable video generation
- video editing
- dreammotion
- segmentation map
- 3d generation
- BOJ
- 논문리뷰
- diffusion model
- video generation
- 네이버 부스트캠프 ai tech 6기
- controlnext
- diffusion models
- 프로그래머스
- diffusion
- transformer
- Python
- 3d editing
- masactrl
- score distillation
- emerdiff
- magdiff
- DP
- visiontransformer
- Today
- Total
목록AI/3D (9)
평범한 필기장
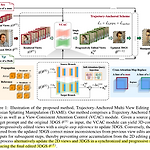
https://arxiv.org/abs/2407.02034 TrAME: Trajectory-Anchored Multi-View Editing for Text-Guided 3D Gaussian Splatting ManipulationDespite significant strides in the field of 3D scene editing, current methods encounter substantial challenge, particularly in preserving 3D consistency in multi-view editing process. To tackle this challenge, we propose a progressive 3D editing strategy tarxiv.org1. I..
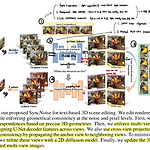
https://arxiv.org/abs/2406.17396 SyncNoise: Geometrically Consistent Noise Prediction for Text-based 3D Scene EditingText-based 2D diffusion models have demonstrated impressive capabilities in image generation and editing. Meanwhile, the 2D diffusion models also exhibit substantial potentials for 3D editing tasks. However, how to achieve consistent edits across multiplearxiv.org1. Introduction I..
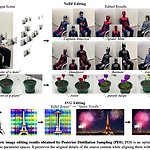
https://posterior-distillation-sampling.github.io/ Posterior Distillation SamplingWe introduce Posterior Distillation Sampling (PDS), a novel optimization method for parametric image editing based on diffusion models. Existing optimization-based methods, which leverage the powerful 2D prior of diffusion models to handle various parametrposterior-distillation-sampling.github.io1. Introduction Edi..
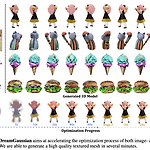
https://arxiv.org/abs/2309.16653 DreamGaussian: Generative Gaussian Splatting for Efficient 3D Content CreationRecent advances in 3D content creation mostly leverage optimization-based 3D generation via score distillation sampling (SDS). Though promising results have been exhibited, these methods often suffer from slow per-sample optimization, limiting their practiarxiv.org1. Introduction 최근 3D ..
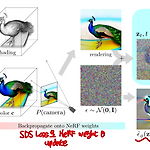
https://arxiv.org/abs/2209.14988 DreamFusion: Text-to-3D using 2D DiffusionRecent breakthroughs in text-to-image synthesis have been driven by diffusion models trained on billions of image-text pairs. Adapting this approach to 3D synthesis would require large-scale datasets of labeled 3D data and efficient architectures for denoiarxiv.org1. Introduction Diffusion model은 다양한 다른 modality에서 적용되는데 성..
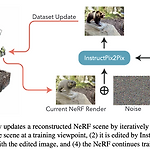
https://arxiv.org/abs/2303.12789 Instruct-NeRF2NeRF: Editing 3D Scenes with InstructionsWe propose a method for editing NeRF scenes with text-instructions. Given a NeRF of a scene and the collection of images used to reconstruct it, our method uses an image-conditioned diffusion model (InstructPix2Pix) to iteratively edit the input images whiarxiv.org1. Introduction NeRF와 같은 3D reconstruction 기술..
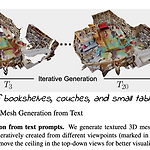
https://arxiv.org/abs/2303.11989 Text2Room: Extracting Textured 3D Meshes from 2D Text-to-Image ModelsWe present Text2Room, a method for generating room-scale textured 3D meshes from a given text prompt as input. To this end, we leverage pre-trained 2D text-to-image models to synthesize a sequence of images from different poses. In order to lift these outparxiv.org요약다루는 task : 2D Text-to-Image m..
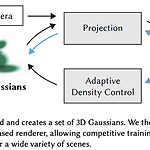
https://arxiv.org/abs/2308.04079 3D Gaussian Splatting for Real-Time Radiance Field RenderingRadiance Field methods have recently revolutionized novel-view synthesis of scenes captured with multiple photos or videos. However, achieving high visual quality still requires neural networks that are costly to train and render, while recent faster methoarxiv.org1. Introduction NeRF 기반의 방식들은 high quali..
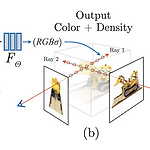
https://arxiv.org/abs/2003.08934 NeRF: Representing Scenes as Neural Radiance Fields for View SynthesisWe present a method that achieves state-of-the-art results for synthesizing novel views of complex scenes by optimizing an underlying continuous volumetric scene function using a sparse set of input views. Our algorithm represents a scene using a fully-conarxiv.org 랩실 인턴을 시작하기 전에 연구 주제에 대한 레퍼런스..