일 | 월 | 화 | 수 | 목 | 금 | 토 |
---|---|---|---|---|---|---|
1 | 2 | 3 | 4 | 5 | ||
6 | 7 | 8 | 9 | 10 | 11 | 12 |
13 | 14 | 15 | 16 | 17 | 18 | 19 |
20 | 21 | 22 | 23 | 24 | 25 | 26 |
27 | 28 | 29 | 30 |
- inversion
- transformer
- 네이버 부스트캠프 ai tech 6기
- memorization
- flipd
- 3d generation
- diffusion models
- segmenation map generation
- rectified flow
- flow matching
- 프로그래머스
- 3d editing
- Python
- Vit
- DP
- BOJ
- 논문리뷰
- masactrl
- image editing
- video generation
- noise optimization
- 코테
- diffusion model
- diffusion
- video editing
- segmentation map
- 코딩테스트
- Programmers
- visiontransformer
- VirtualTryON
- Today
- Total
평범한 필기장
[평범한 학부생이 하는 논문 리뷰] InitNO : Boosting Text-to-Image Diffusion Models via Initial Noise Optimization (CVPR 2024) 본문
[평범한 학부생이 하는 논문 리뷰] InitNO : Boosting Text-to-Image Diffusion Models via Initial Noise Optimization (CVPR 2024)
junseok-rh 2025. 3. 17. 18:02Paper : https://arxiv.org/abs/2404.04650
InitNO: Boosting Text-to-Image Diffusion Models via Initial Noise Optimization
Recent strides in the development of diffusion models, exemplified by advancements such as Stable Diffusion, have underscored their remarkable prowess in generating visually compelling images. However, the imperative of achieving a seamless alignment betwe
arxiv.org
Abstract
모든 random noise가 의미적으로 faithful한 이미지를 생성하는데 효과적이지 않다. 본 논문에서는 cross-attention response score와 self-attention conflict score를 디자인해 initial noise를 평가하고, 이를 이용해 noise를 optimization하는 InitNO를 제안한다.
1. Introduction
거대한 text-image dataset으로 T2I diffusion model을 학습함에도 불구하고, 주어진 text prompt와 정확히 align된 이미지를 생성하는 것에는 한계가 있다. 아래와 같은 issue들이 존재한다.
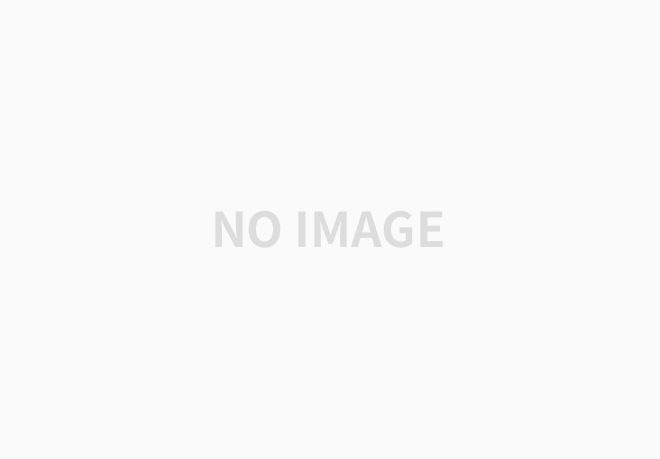
이러한 문제점들이 본 논문에서는 유효하지 않은 initial noise의 존재라고 여긴다.
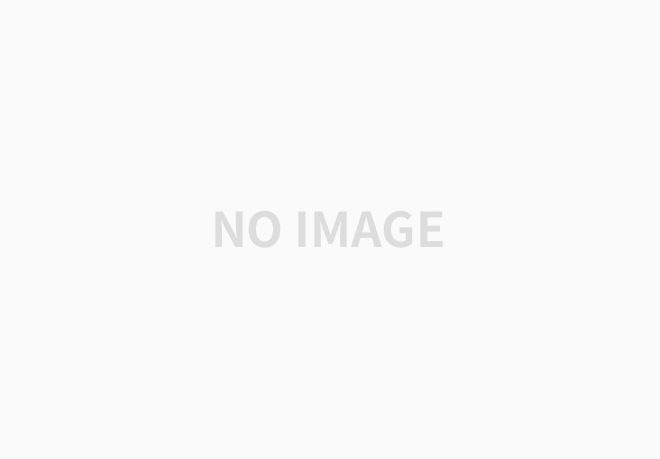
위에서 볼 수 있듯이, 모든 random noise가 visually-consistent image를 생성할 수 있는 것은 아니다. Initial noise는 vaild와 invalid region으로 나뉠 수 있다. 본 논문의 목적은 initial noise를 vaild region으로 옮겨서, input prompt와 충실히 align된 visually-coherent 이미지의 생성을 용이하게 하는 것이다.
Cross-attention map은 text와 image feature 사이의 관계를 capture하고, self-attention map은 image feature 안의 공간적인 위치 사이의 관계를 capture한다. 이러한 map들은 subject neglect와 subject mixing을 효과적으로 측정한다. 이러한 map들을 이용해, 본 논문은 cross-attention response score와 self-attention conflict score를 디자인한다. Score threshold를 셋팅함으로써, inital noise space의 partitioning을 달성한다. 본 논문에서는 subject의 정확한 rendering이 정확한 attribute binding을 조성한다는 것을 발견한다.
Initial noise navigation에서의 주요 challenge는 noise의 under-optimization과 over-optimization사이의 밸런스를 맞추는 것이다. Under-optimization은 misalignment, over-optimization은 initial distribution으로부터 멀어져 distorted image를 야기하는 위험을 가진다. 이를 해결하기 위해, 본 논문은 carefully-crafted noise optimization pipeline을 제안한다. Inital latent space의 명확한 모델링을 가지고, 새로운 distribution alignment loss는 initial distribution으로 optimized noise가 향하도록 보장하기 위해 통합된다.
2. InitNO
Initial Noise Optimization(InitNO)를 제안한다. 이는 initial noise latent space partitioning과 noise optimization pipeline을 통합한다.
2.1 Initial latent space partitioning
본 논문은 cross-attention map과 self-attention map이 subject neglect과 subject mixing을 각각 측정할 수 있다고 주장한다. 이러한 map들을 활용해서, 본 논문은 cross-attention response score와 self-attention conflict score를 formulate한다. Score threshold를 설정함으로써, initial latent space의 partitioning을 달성한다.
Cross-attention response score
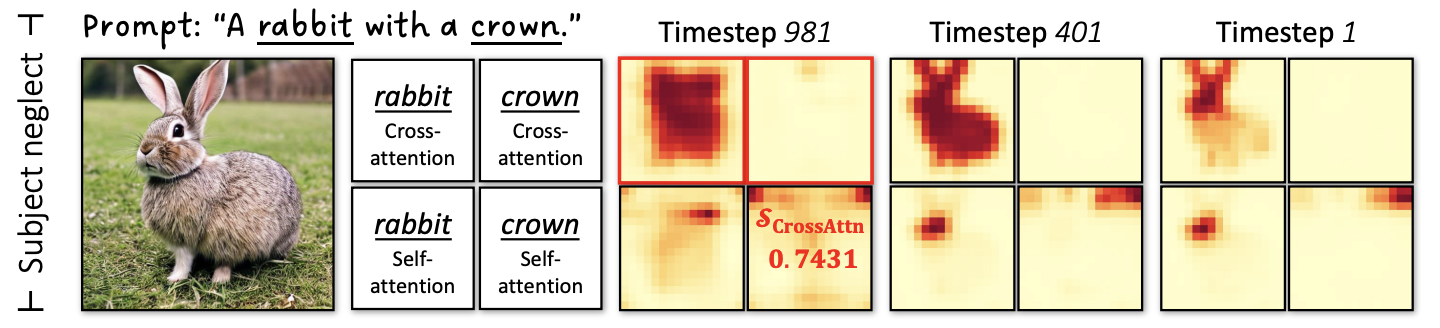
Proficient denoising process는 early stage동안 각 subject에 대한 충분히 높은 high cross-attention response를 할당할 수 있다. 역으로, 낮은 attention response는 target subject의 부재를 야기할 수 있다. 본 논문에서는 cross-attention response score를 다음과 같이 정의한다.

Y는 target token들의 set을 나타낸다. 특히 본 논문에서는 SCrossAttn은 initial noise와 첫 denoising step에 대해서 계산된다. 본 논문에서는 threshold를 τc=0.2로 설정해서 이보다 낮으면 valid, 아니면 invalid로 분류한다.
Self-attention conflict score
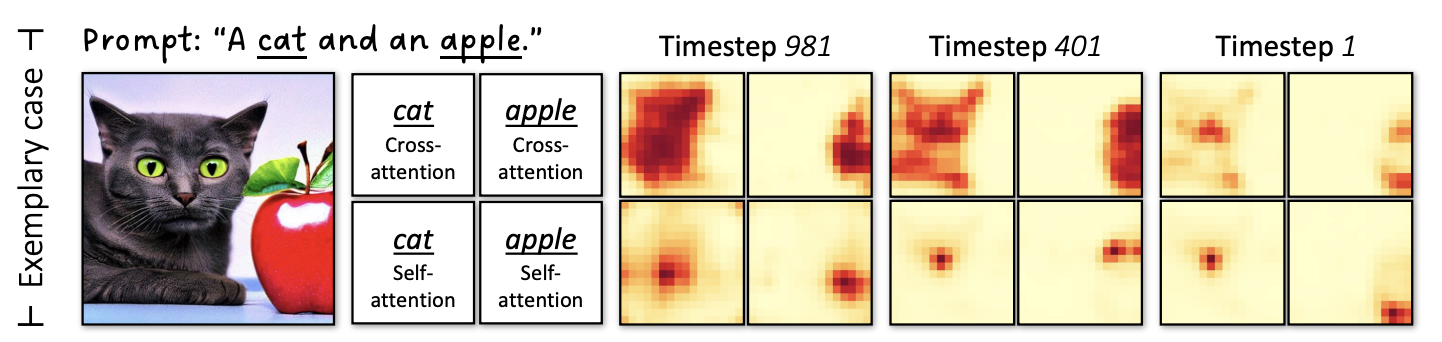
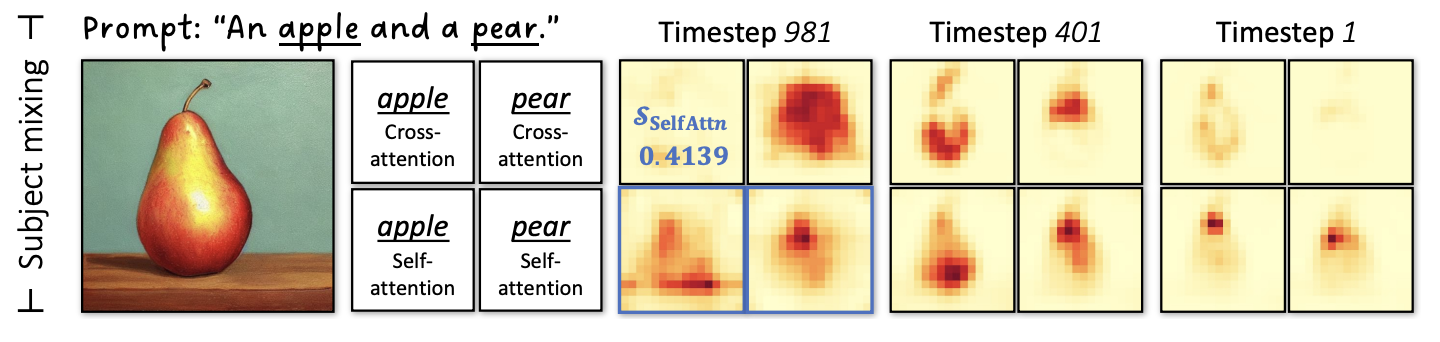
Subject token yi에 대해서 먼저 maximum cross-attention value에 대응되는 공간적인 coordinate (xi,yi)를 query한다.

그 후에, 그에 대응되는 self-attention map Acxi,yi를 얻는다. 모범 사례 denoising process에 대해서, 다른 subject token의 self-attention maps은 spatial domain안에서 명확히 나눠질 수 있다. 그러나 기존 diffusion models은 self-attention map overlap 문제가 발생한다. 이는 subject mixing 문제를 야기한다. 이를 측정하기 위해서, self-attention conflict score를 도입한다.

SSelfAttn은 initial noise와 첫 denoising step을 기반으로 계산된다. Threshold τs=0.3로 설정한다. τs 아래면 valid로, 아니면 invalid로 판별된다.
최종적으로, 두 score 모두가 threshold보다 낮으면 valid noise로, 아니면 invalid noise로 판별된다.
2.2 Noise optimization pipeline
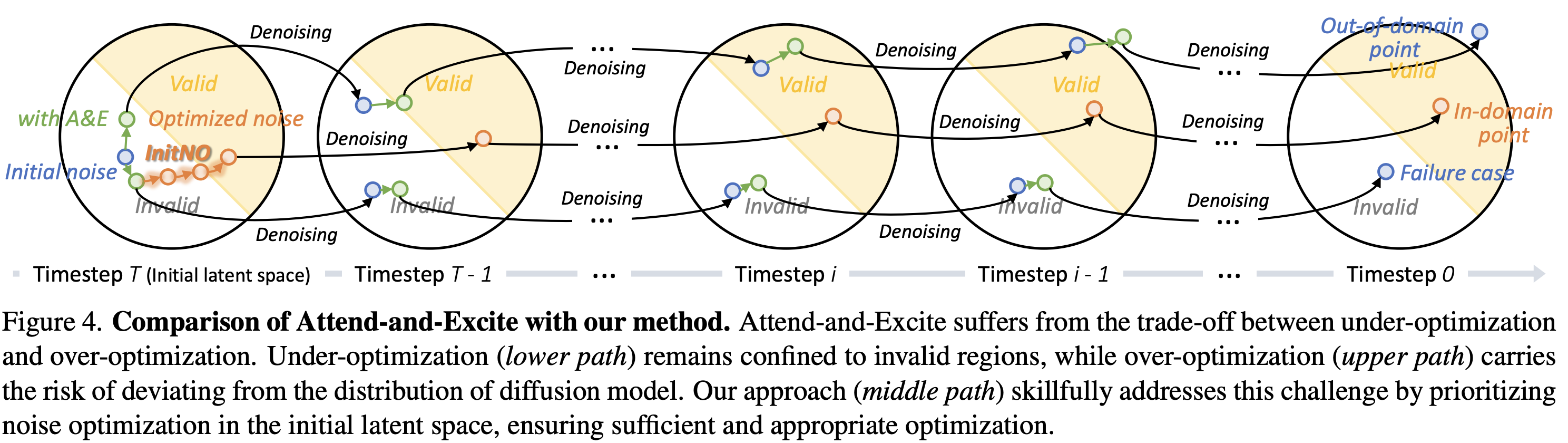
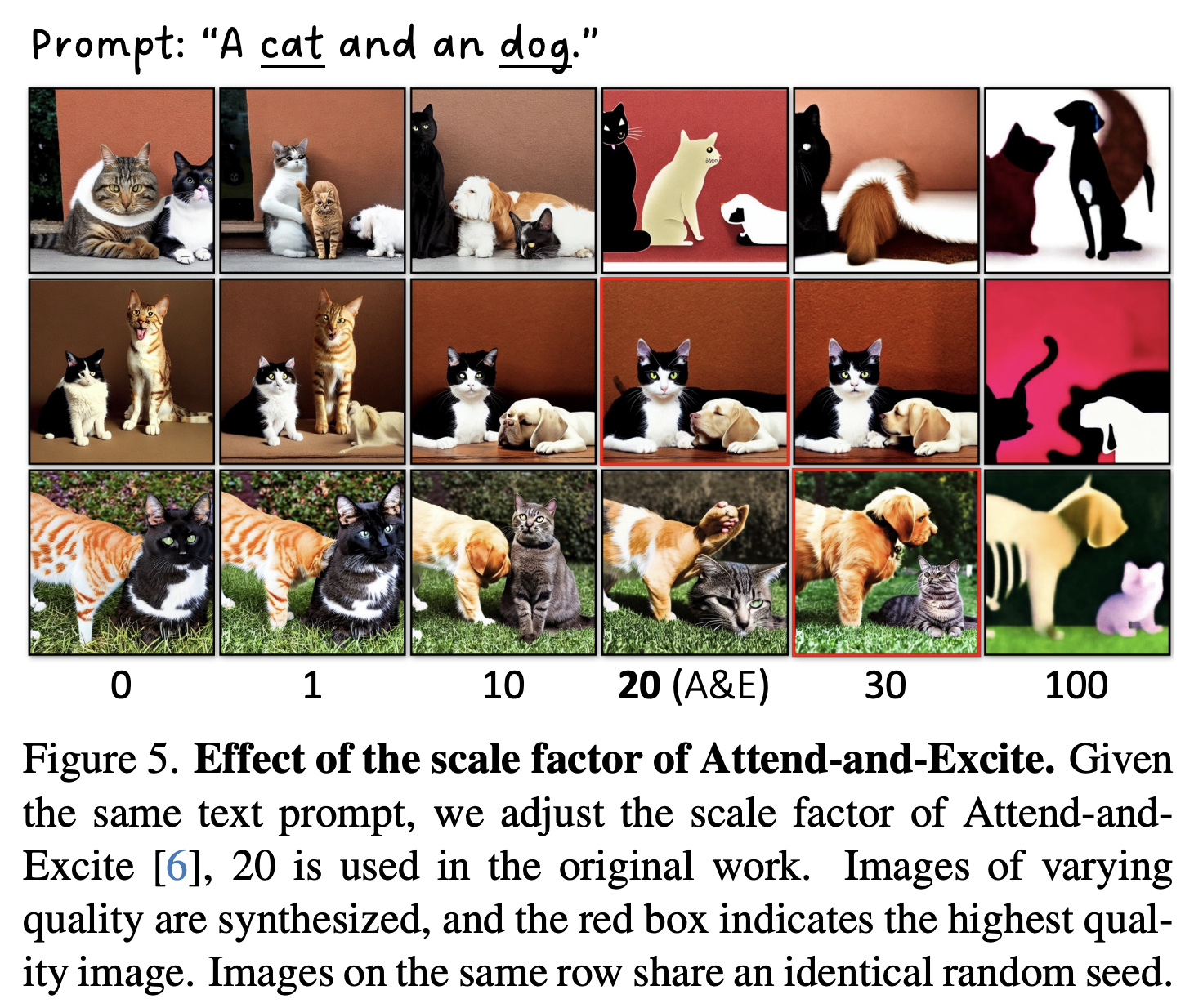
기존의 방식(Attend-and-Excite)는 정밀한 파라미터 조정이 필요하고, 예시마다 적당한 파라미터가 존재한다. 또한 under-optimization과 over-optimization문제가 발생한다. 이는 각각 subpar quality와 out-of-domain 문제를 발생시킨다.
InitNO는 initial noise를 valid region에서 random sampled noise로 이동시키고, Intial noise가 standard Gaussian distribution을 준수하도록 한다.
Noise Update Strategy
기존의 방식과 다르게 본 논문은 다음과 같은 optimization strategy를 채택한다.

μ,σ는 0,1로 초기화된 Gaussian distribution의 평균과 표준편차이다.
ϵ′←μ′+σ′ϵ, ϵ′∼N(μ′,σ′2)
Loss Function
Target Gaussian distribution N(μ,σ2)와 standard Gaussian distribution N(0,1)사이의 consistency를 제한하기 위해서 KL divergence를 distribution alignment loss로 활용한다.

최종 loss function은 다음과 같다.

LCrossAttn=SCrossAttn,LSelfAttn=SSelfAttn
λ1=1,λ2=1,λ3=500
Noise optimization procedure
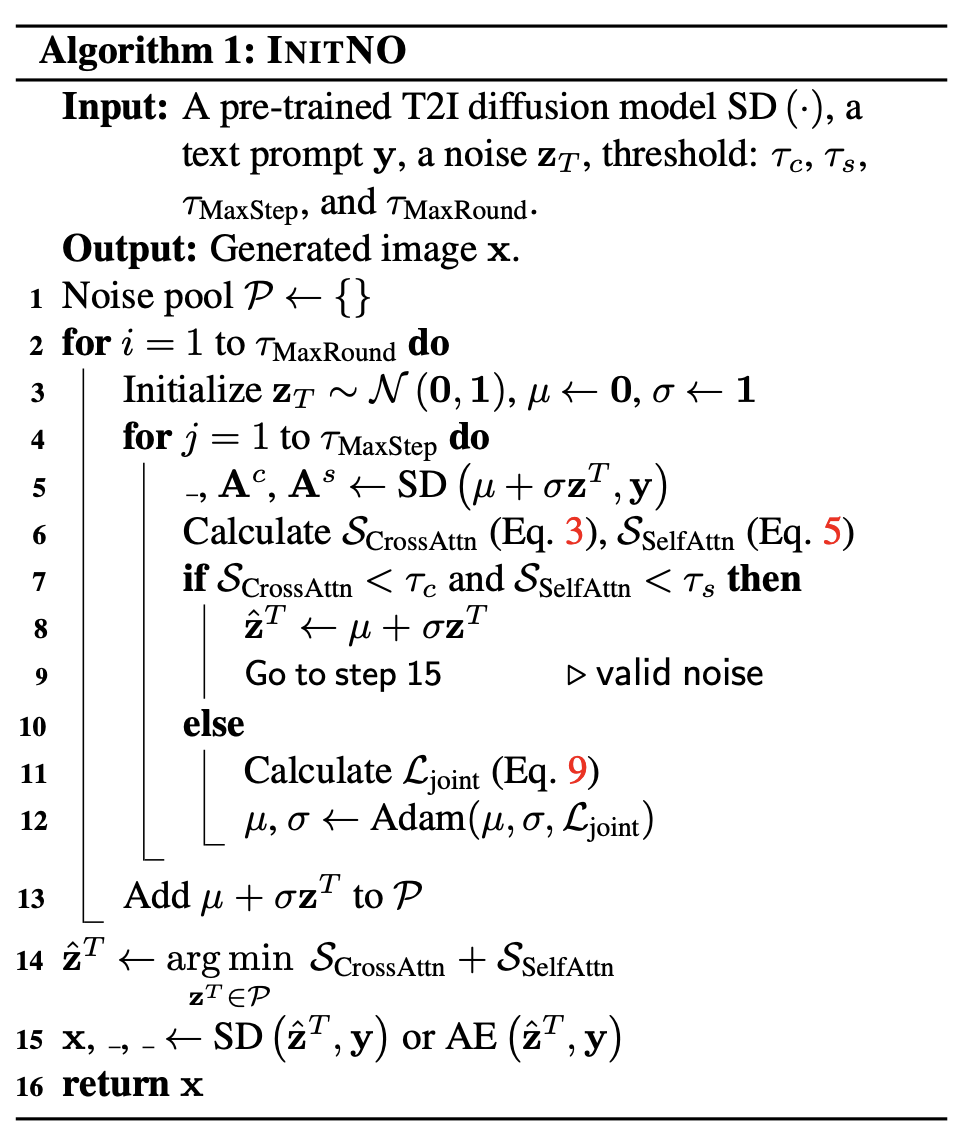
τMaxStep로 optimization iteration을 제한한다. 이 과정동안 vaild region에 도달하는데 실패하면, zT를 다시 샘플링하고 μ,σ를 reset해서 다시 반복한다. 이 과정도 τMaxRound로 제한한다. τMaxStep,τMaxRound는 50, 5로 둔다. 이 과정에서 적당한 노이즈를 찾지 못하면 존재하는 noise pool P에서 SCrossAttn,SSelfAttn의 합을 minimize하는 noise를 선택한다.
3. Experiments
3.1 Qualitative Comparison
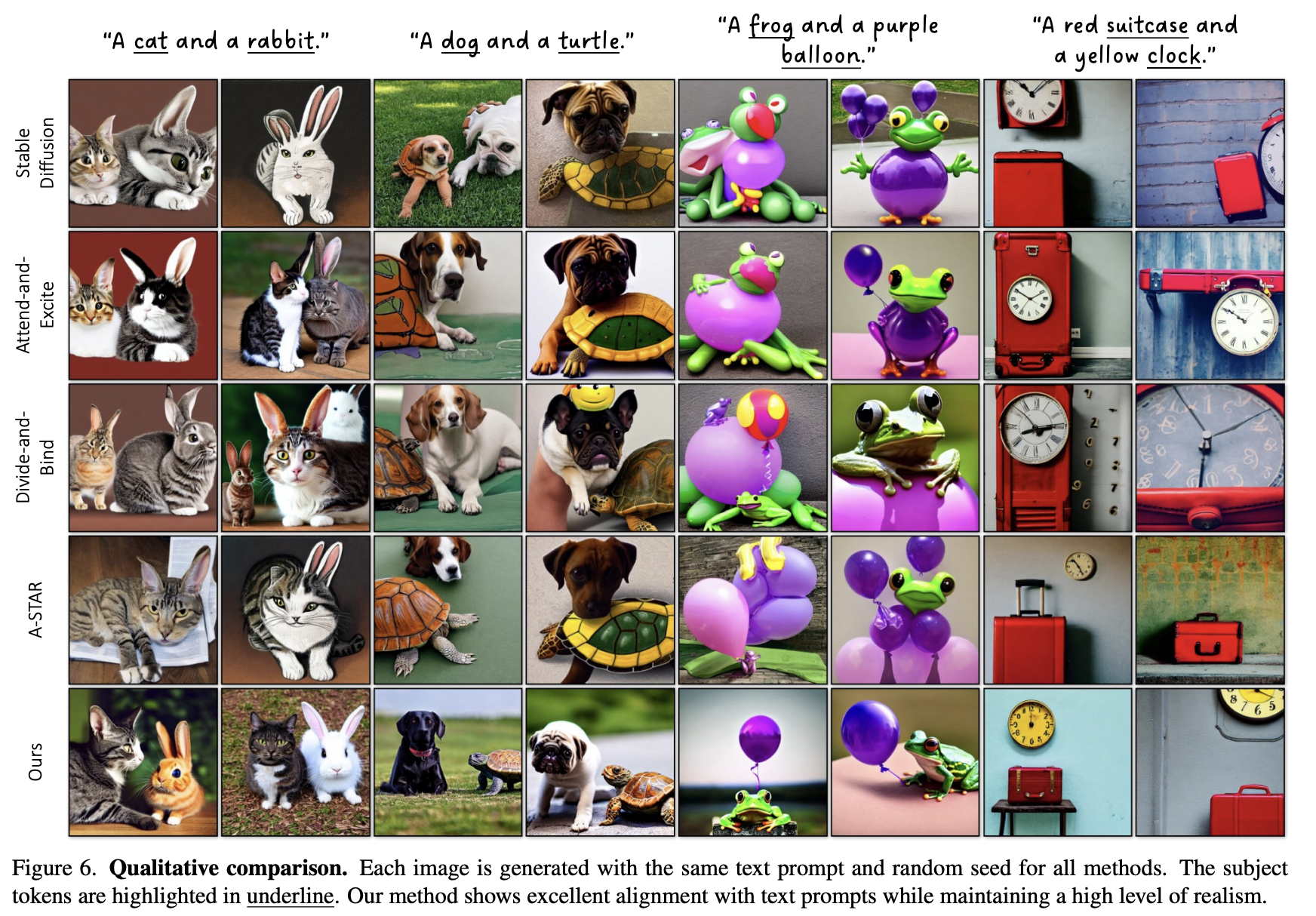
3.2 Quantitative comparison
Objective Evaluation

User Study
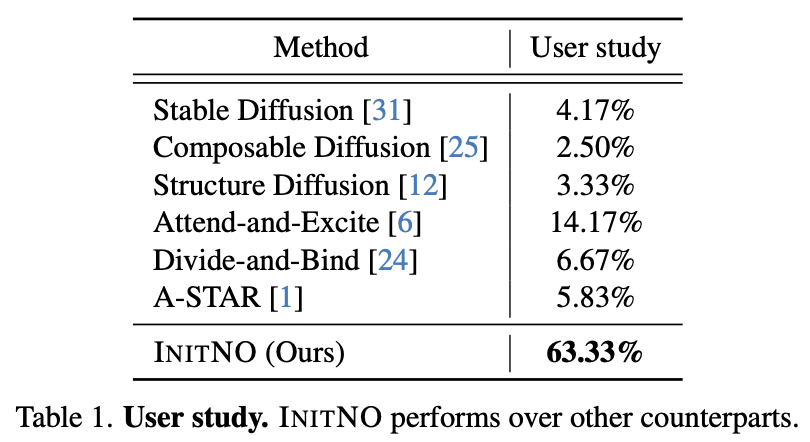
3.4 Ablation Study
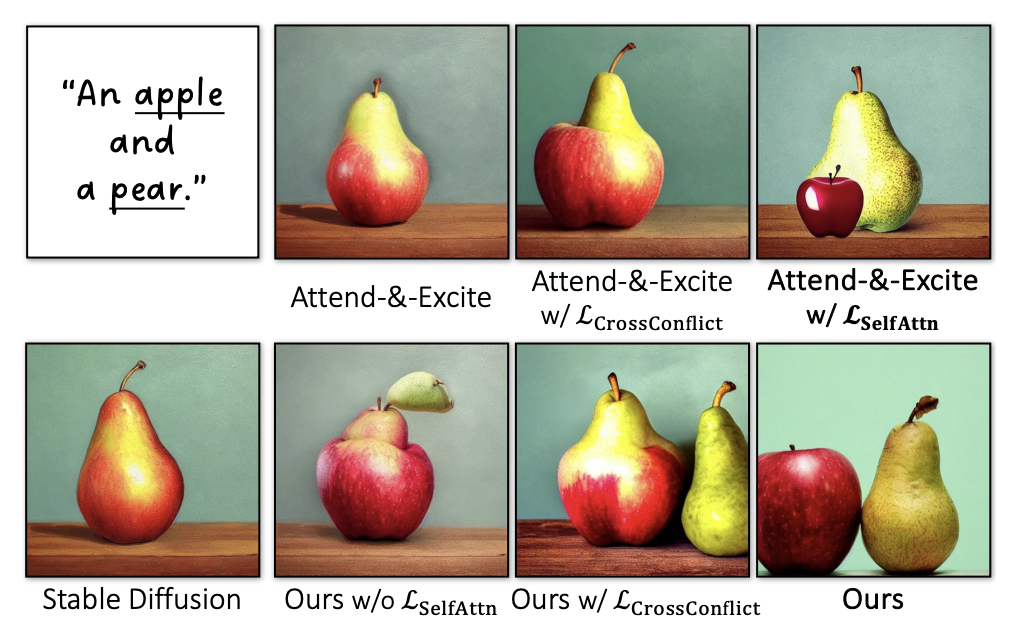
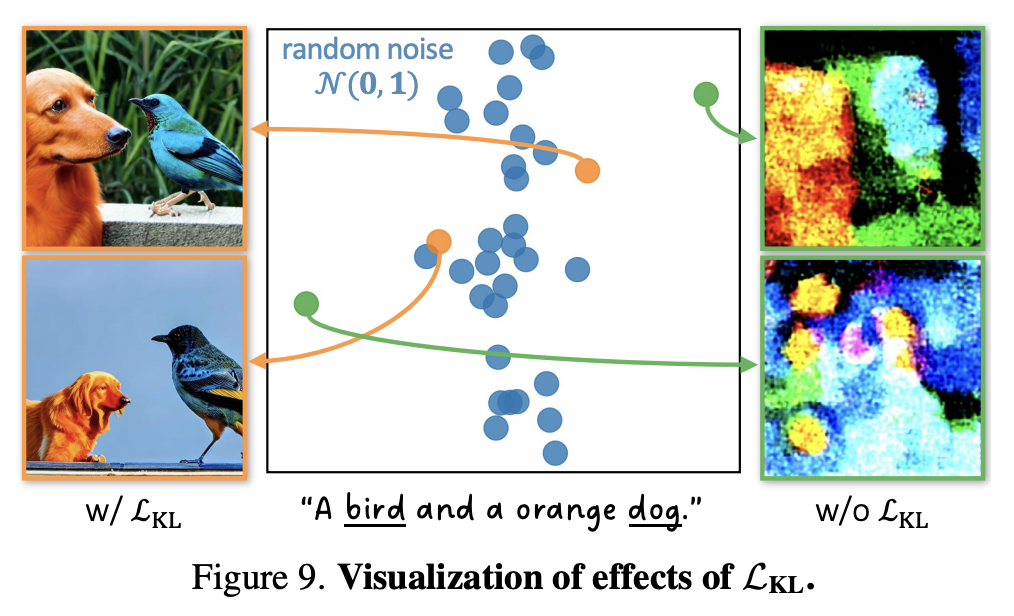
3.5 Grounded Text-to-Image
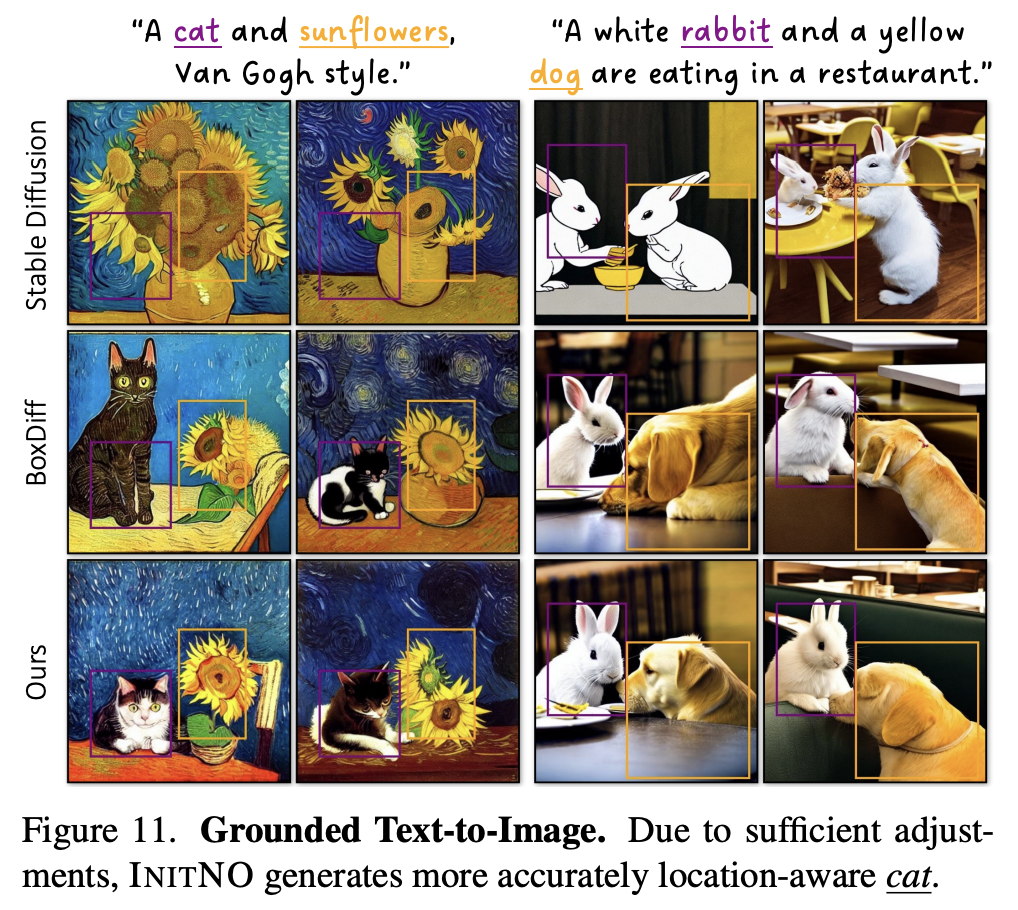
3.6 More Results
Complex prompts
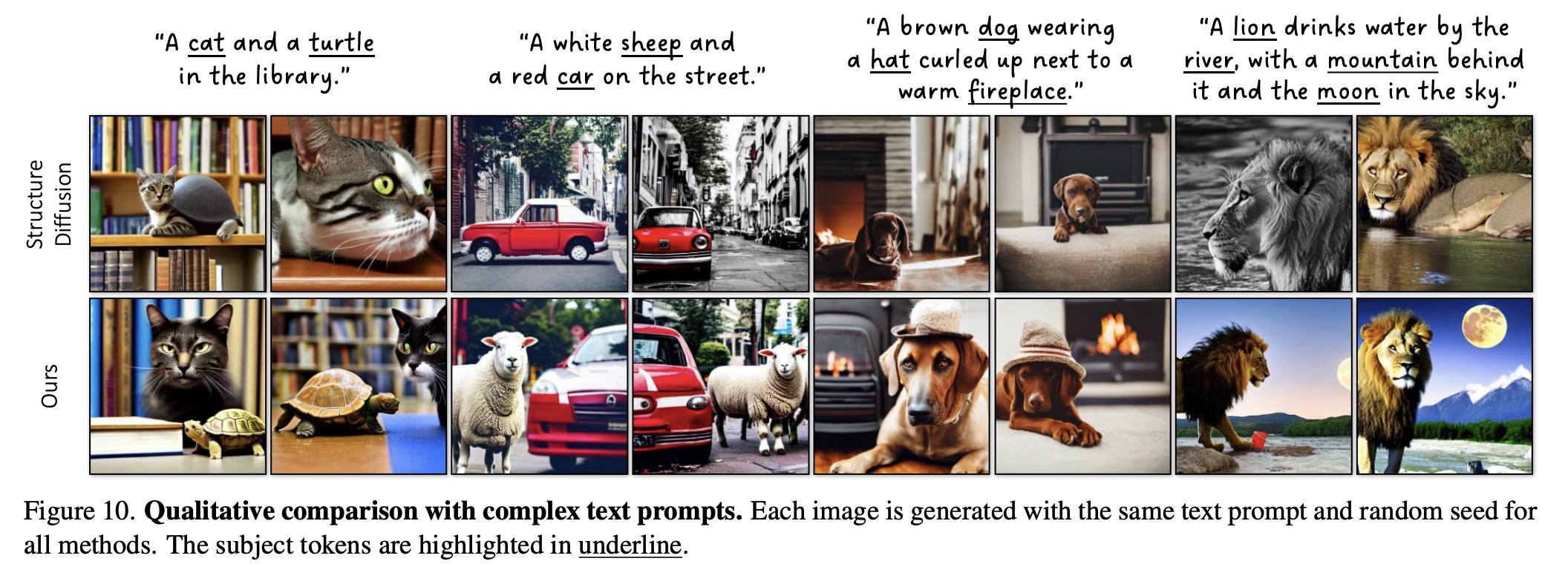
Visualization of the attention maps
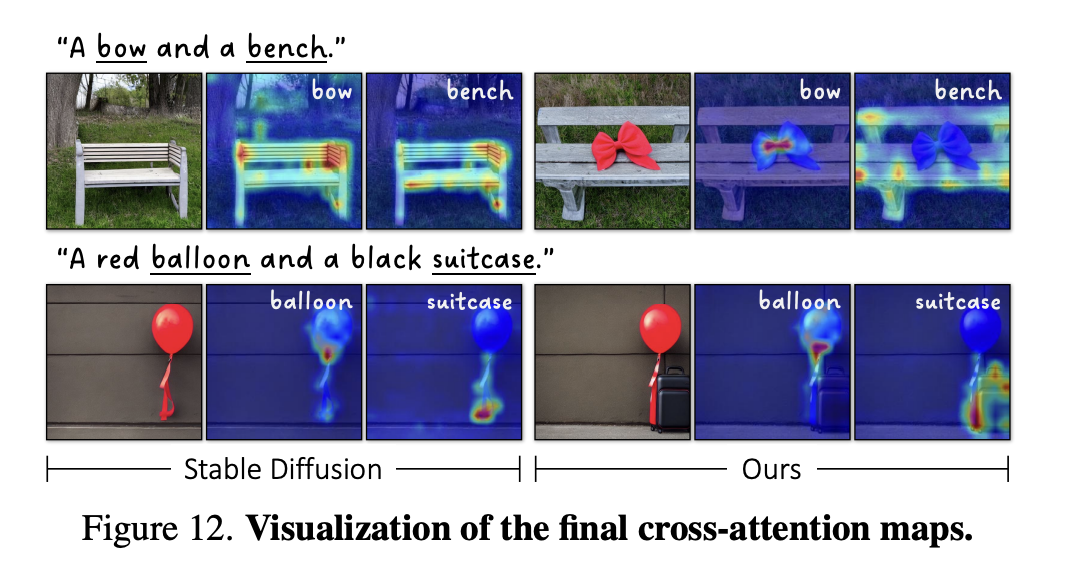