일 | 월 | 화 | 수 | 목 | 금 | 토 |
---|---|---|---|---|---|---|
1 | ||||||
2 | 3 | 4 | 5 | 6 | 7 | 8 |
9 | 10 | 11 | 12 | 13 | 14 | 15 |
16 | 17 | 18 | 19 | 20 | 21 | 22 |
23 | 24 | 25 | 26 | 27 | 28 |
- video generation
- video editing
- diffusion model
- 네이버 부스트캠프 ai tech 6기
- 코딩테스트
- Programmers
- dreammotion
- VirtualTryON
- rectified flow
- 코테
- visiontransformer
- 3d editing
- 논문리뷰
- masactrl
- flow matching
- emerdiff
- DP
- segmenation map generation
- image editing
- score distillation
- transformer
- diffusion
- Vit
- controlnext
- Python
- 프로그래머스
- 3d generation
- diffusion models
- BOJ
- segmentation map
- Today
- Total
목록2025/02 (2)
평범한 필기장
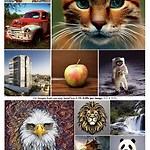
Paper : https://arxiv.org/abs/2309.06380 InstaFlow: One Step is Enough for High-Quality Diffusion-Based Text-to-Image GenerationDiffusion models have revolutionized text-to-image generation with its exceptional quality and creativity. However, its multi-step sampling process is known to be slow, often requiring tens of inference steps to obtain satisfactory results. Previous attemparxiv.orgAbstr..
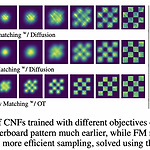
Paper : https://arxiv.org/abs/2210.02747 Flow Matching for Generative ModelingWe introduce a new paradigm for generative modeling built on Continuous Normalizing Flows (CNFs), allowing us to train CNFs at unprecedented scale. Specifically, we present the notion of Flow Matching (FM), a simulation-free approach for training CNFs basearxiv.org(성민혁 교수님 강의 자료 참고 : https://www.youtube.com/watch?v=B4F..